As the world entered the age of big data, the need for its storage also increased. Until 2010, this was a major challenge and concern for the enterprise industry. The main focus was on creating a framework and solutions for storing data.
Now that Hoodoop and other architectures have successfully resolved the storage issue, the focus has shifted to the processing of this data. Data science is the secret sauce here. Everything you see in Hollywood sci-fi movies will come true with data science. The future of artificial intelligence is data science. Therefore, it is important to understand what Data science is and how it can add value to your business.
In this blog following topics will be covered
- Why Data Science?
- Who is a Data Scientist?
- What does a Data Scientist do?
- How is it different from Business Intelligence (BI) and Data Science?
- The lifecycle of Data Science with the help of a use case
Do you want to become a Data Science Expert join the Live Training Program on Naresh I Technology .
What is Data Science?
Data science is the process of combining experts, programming skills, knowledge of mathematics, and statistics from the field to gain meaningful insights from data. Data science practitioners use machine learning algorithms for numbers, text, images, video, audio, and more to develop artificial intelligence (AI) systems to perform tasks required by the humanities. These systems generate statistics that can be translated into clear business value for the consequences and for business users.
Data science professionals are emerging as one of the most promising and desirable career paths for skilled professionals. Successful data professionals today realize that large-scale data overrides traditional skills for analyzing data
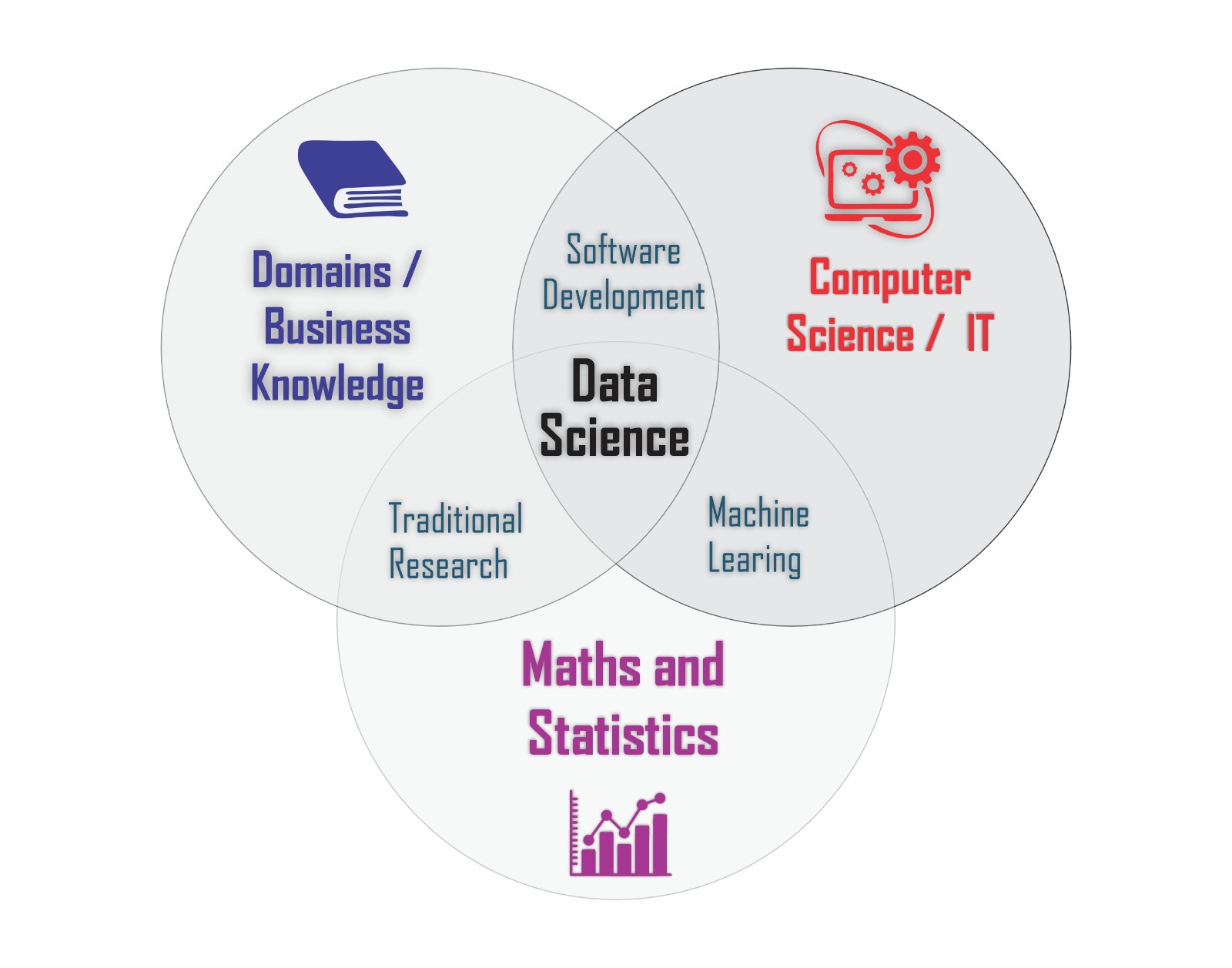
processing and programming skills. In order to find effective statistics for their organizations, data scientists must have mastered the entire spectrum of the Data science life cycle and have the flexibility and understanding to maximize revenue at each stage of the process.
More and more companies are coming to understand the importance of Data science, AI and machine learning. Companies that want to compete in the big data age regardless of industry or size need to effectively develop and implement Data science skills or risk-levying skills.
Looking for a Data Science Mock Interview Test Join Naresh I Technology.
Who is a data scientist?
Data scientists have several definitions. Simply put, a data scientist is someone who adheres to the art of data science. The most popular term for ‘data scientist’ is Created by Patil and Jeff Hamperpatcher.
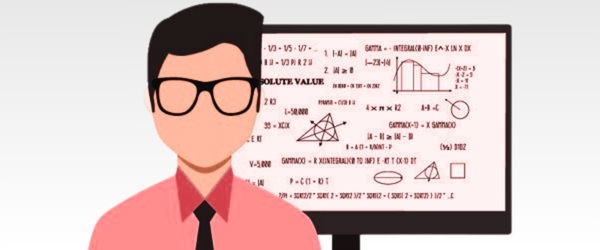
Data scientists are people who twist complex data problems with strong expertise in certain fields of science. They work with a number of components, including mathematics, statistics, and computer science (although not experts in these fields).
Looking for a Data Science Mock Interview Test Join Naresh I Technology.
What does a Data Scientist do?
The role of the data scientist is really a challenge! Although the skill-packages and capabilities used by data scientists may vary as a skilled data scientist, he:
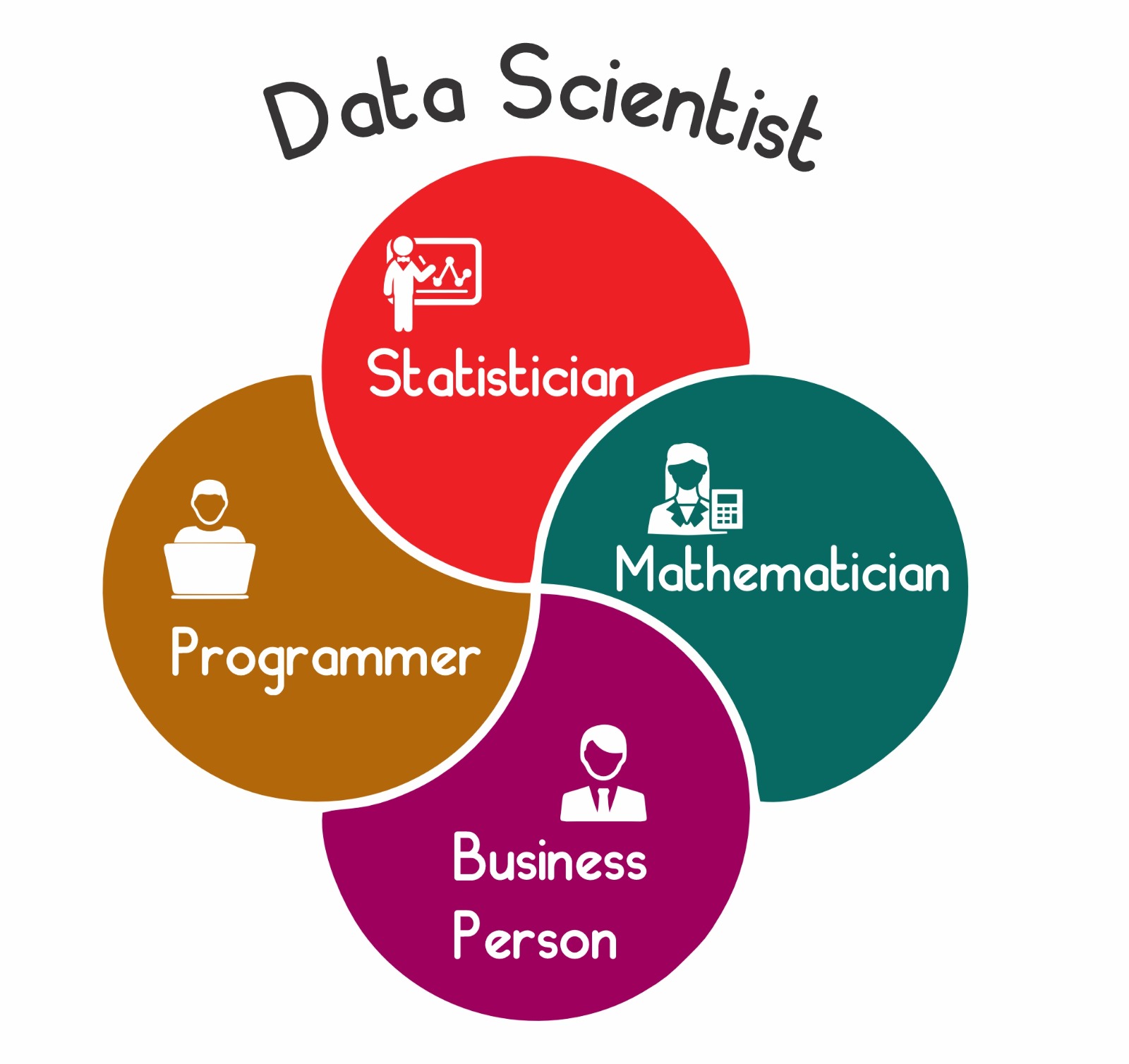
- Be very innovative and unique in his approach to extracting data, gaining useful insights into solving business problems and challenges, and using various technologies intelligently.
- Ability to find and create rich data sources.
- A handful of experience in data mining techniques such as graph analysis, method finding, result perspectives, clustering or statistical analysis.
- Develop working models, systems, and tools using experimental and functional methods and techniques.
- Analyze data from different sources and perspectives and find hidden statistics.
- Conditioning data - that is, converting data into an effective format using statistics, mathematical tools, and forecast analysis.
- Research, analyze, implement, and present statistical methods to obtain practical statistics.
- Manage large amounts of data between hardware, software, and bandwidth limitations.
- Create visualizations that help anyone easily understand the trends of data analysis.
- Become a team leader and interact effectively with other business analysts, product managers and engineers.
Do you want to become a Data Science Expert join the Live Training Program on Naresh I Technology.
How is it different from Business Intelligence (BI) and Data Science?
Data Science:
-
- Data science is the practice of extracting information and knowledge from data using a variety of scientific methods, techniques, and processes.
- It is defined as a combination of various mathematical tools, algorithms, statistics, and machine learning techniques that can be used to find hidden patterns and statistics from the data that support the decision-making process.
- Data science deals with both structured and unstructured data. This is relevant for data processing and large data.
- Data science involves studying historical trends so that its results are subject to redefining current trends and predicting future trends.
Business Intelligence:
-
- Business Intelligence (BI) is basically a set of technologies, applications, and processes used by companies to analyze business data.
- It is basically used to turn raw data into meaningful information, which is used for business decision making and profitable activities.
- It deals with the analysis of structured and sometimes unstructured data, leading to new and profitable business opportunities. It supports factual decision making rather than hypothetical decision making.
- Thus it directly influences the business decisions of a company. Business intelligence tools improve a company's chances of entering a new market and help them study the impact of marketing efforts.
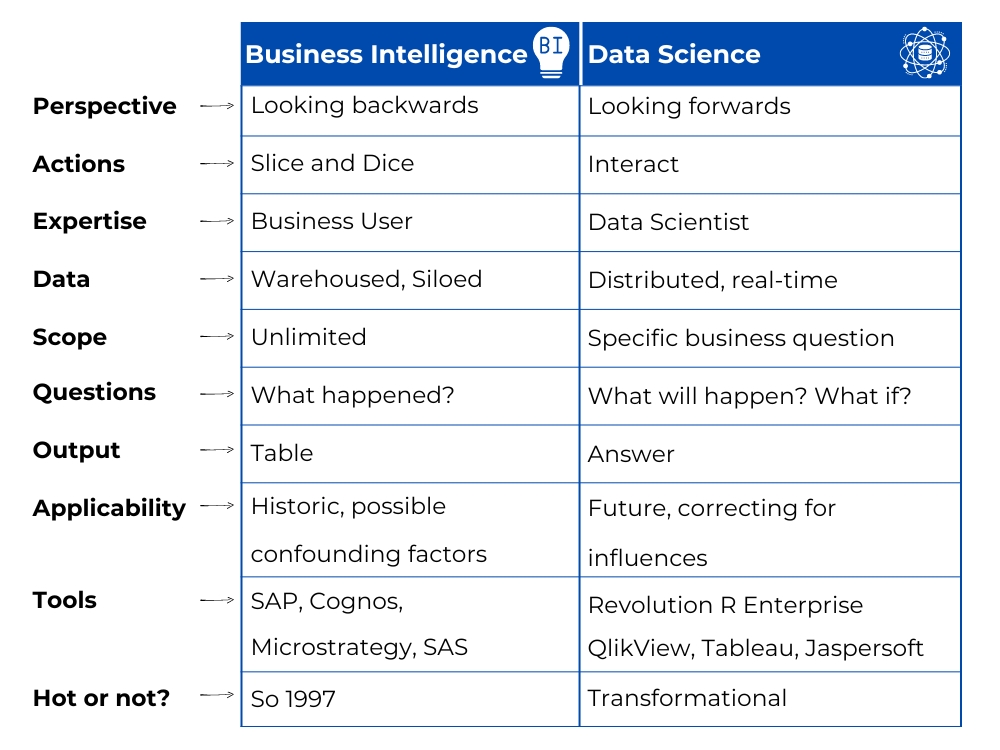
Looking for a Data Science Mock Interview Test Join Naresh I Technology.
The lifecycle of Data Science with the help of a use case
The main stages of the data science life cycle are given below:
-
Discovery:
-
The first step is discovery, which involves asking the right question. When you start any data science project,
you need to determine what the basic requirements, priorities and project budget are.
- At this point, we need to determine all the requirements such as project number, technology, time, data, and an end goal, and then the business problem can be designed at the first conceptual level.
- Data preparation:
- Data preparation is also known as data munching. At this point, we need to do the following:
- Data cleaning
- Data reduction
- Data integration
- Data transformation
- After completing all of the above tasks, we can easily apply this data to our additional processes.
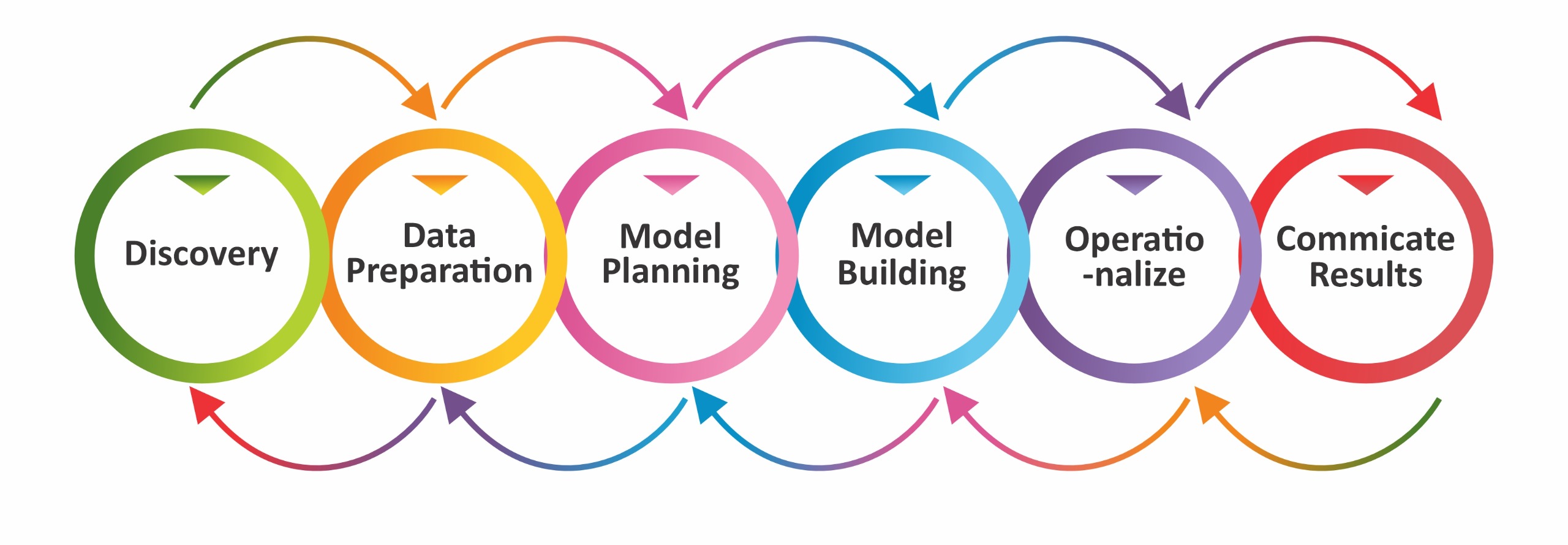
-
Model planning:
- At this stage, we need to determine the different methods and techniques for establishing the relationship between the input variables.
- We use research data analysis (EDA) using various statistical formulas and visualization tools to understand the relationships between variables and to see what data can tell us.
-
Model Building:
- At this stage, the model construction process begins.
- We will create databases for training and testing purposes.
- We will use various techniques such as association, classification and clustering to create the model.
-
Operationalize:
- At this stage, we will provide the final reports, summaries, code and technical documentation of the project.
- This step gives you a brief overview of the full project performance and other factors prior to full deployment.
-
Communicate the results:
- At this stage, we will check whether we are reaching the goal we set in the initial stage.
- We will communicate the findings and final results with the business team.
USE CASE - Amazon
- Amazon has worked hard to become a customer-centric platform. Amazon Forecast relies heavily on analytics to increase customer satisfaction.
- This is done through a customized referral system.
- This referral system is a hybrid type that includes cooperative purification, which is widespread in nature.
- Analyzes Amazon user historic purchases to recommend more products.
- It also provides suggestions from other users who use similar products or give similar ratings.
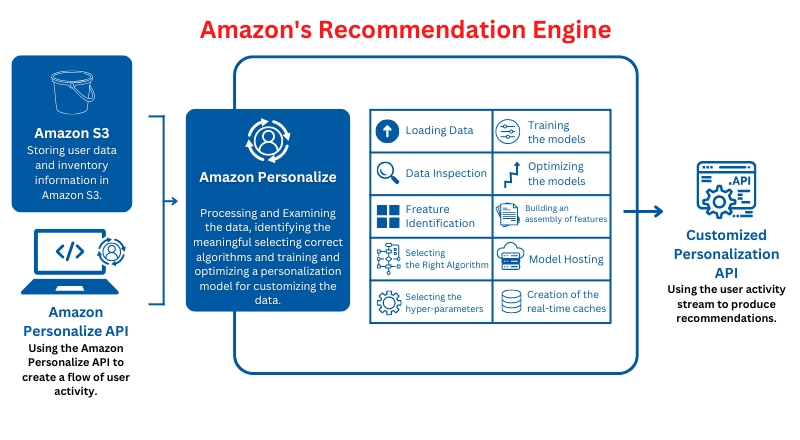
- Amazon has an expected shipping model that uses big data to predict which products users will buy.
- It analyzes your purchasing system and sends products you may use in the future to your nearest warehouse.
- Amazon upgrades prices on its websites with various parameters in mind such as user performance, order history, prices offered by competitors and product availability.
- With this method, Amazon offers discounts on popular products and profits on less popular products.